In recent years, machine learning (ML) has emerged as a powerful tool in medical research. As a subset of artificial intelligence, ML allows computers to learn from data and make predictions or decisions without being explicitly programmed. The potential of ML in medical research is vast, from diagnosing diseases to predicting treatment outcomes. In this article, we will explore the role of machine learning in medical research, its importance, and some of the critical applications of this technology in healthcare.
Applications of Machine Learning in Medical Research
- Drug Discovery: Machine learning algorithms can assist in the identification and design of potential new drugs for various diseases. This can be done through the analysis of large datasets to find patterns and correlations that can be used to predict drug-target interactions or to generate new molecules with desired properties.
- Medical Imaging Analysis: Machine learning can be used to analyze medical images, such as X-rays, CT scans, and MRI scans. These algorithms can detect abnormalities and classify images, making diagnoses more efficient and accurate.
- Electronic Health Records Analysis: Machine learning can be used to analyze electronic health records (EHRs) to identify trends and patterns in patient data. This can help predict disease risk and aid in diagnosis and treatment decisions.
- Predictive Modeling: Machine learning algorithms can be used to develop predictive models for various medical conditions. For example, machine learning can be used to predict the risk of developing certain diseases, such as cancer or diabetes, based on patient characteristics, lifestyle factors, and medical history. These models can help healthcare providers identify patients at high risk and take preventive measures.
Overall, the application of machine learning in medical research has the potential to revolutionize the way we approach disease diagnosis, treatment, and prevention. By analyzing large and complex datasets, machine learning algorithms can identify patterns and relationships that may be missed by human analysis. This can lead to more accurate diagnoses, personalized treatment plans, and better patient outcomes.
Advantages of Machine Learning in Medical Research
- Faster analysis of data: Machine learning algorithms can quickly analyze large amounts of medical data, including electronic health records, clinical trial data, and medical imaging. This speed of analysis can help researchers to identify patterns and correlations that may not have been apparent with traditional analysis methods.
- Increased accuracy in diagnoses: Machine learning algorithms can be trained on large datasets of patient information to recognize patterns and predict outcomes. This can lead to more accurate diagnoses, allowing doctors to identify illnesses and conditions at earlier stages and improve patient outcomes.
- Customized treatment plans: Machine learning algorithms can help researchers identify patient subgroups based on genetics, lifestyle, and other factors and recommend personalized treatment plans. This helps ensure that patients receive the most effective treatment possible while minimizing the risk of side effects.
- Improved patient outcomes: The use of machine learning in medical research can help to improve patient outcomes, including reduced hospital stays, fewer medical errors, and lower costs. Machine learning algorithms can also help to predict patient outcomes, allowing doctors to take proactive measures to prevent complications.
Overall, the use of machine learning in medical research has the potential to transform the way healthcare is delivered by improving diagnoses, identifying personalized treatment plans, and ultimately leading to better patient outcomes.
Challenges and Limitations of Machine Learning in Medical Research
- Ethical Considerations: Machine learning algorithms must adhere to ethical standards and ensure that patient data privacy is protected. There may also be concerns about the transparency and explainability of the decision-making process of machine learning algorithms.
- Limited Data Availability: Machine learning models require large amounts of data to be effective, but in medical research, it can be challenging to obtain adequate and relevant data. The lack of data can lead to inaccurate results and undermine the reliability of machine learning predictions.
- Need for Interdisciplinary Collaboration: Machine learning in medical research requires a collaborative approach involving healthcare professionals, computer scientists, statisticians, and other experts. Without interdisciplinary collaboration, there is a risk of limited understanding of the data and inadequate model development.
- Technical Challenges: The technical aspects of machine learning can pose challenges in the medical research context. This includes selecting appropriate algorithms, interpreting results, and ensuring the reliability and validity of models. There may also be challenges in integrating machine learning models into clinical practice.
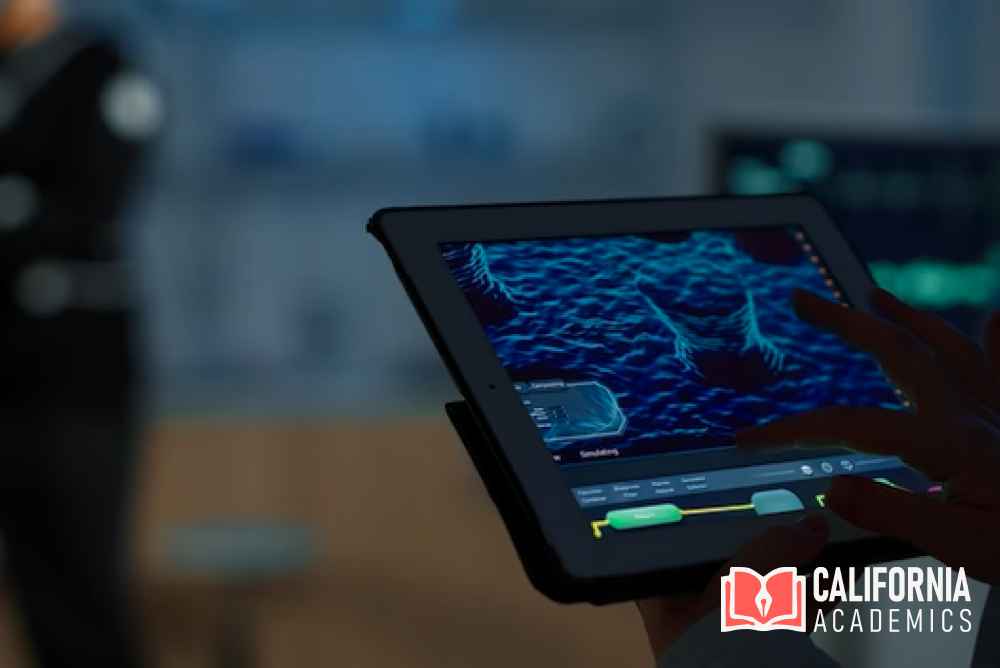
Case Studies of Machine Learning in Medical Research
Machine learning has been utilized in various medical research areas, producing impressive results. Here are some examples of case studies:
- Analysis of Electronic Health Records to Identify Risk Factors for Alzheimer’s Disease: Machine learning algorithms were utilized to analyze electronic health records to identify patterns in patients’ medical histories that may indicate a higher risk for Alzheimer’s disease. The study found that individuals with a history of depression, hypertension, and diabetes were at an increased risk of developing Alzheimer’s disease. This approach has the potential to improve the accuracy of Alzheimer’s disease diagnosis and facilitate early intervention.
- Detection of Cancer Cells in Medical Imaging: Machine learning algorithms have been used to analyze medical images to detect the presence of cancer cells. This approach can help radiologists to identify suspicious areas more accurately, leading to earlier detection and treatment of cancer.
- Identification of Gene Expression Patterns in Cancer Diagnosis: Machine learning algorithms have been applied to analyze gene expression patterns in cancer diagnosis. This approach has the potential to identify novel biomarkers for cancer diagnosis, which may lead to more accurate diagnoses and personalized treatment plans.
These case studies demonstrate the potential for machine learning in medical research to improve the accuracy and efficiency of diagnoses, leading to earlier intervention and improved patient outcomes. However, there are also challenges and limitations that must be addressed, such as the need for interdisciplinary collaboration and the ethical considerations involved in utilizing machine learning in healthcare.
Future of Machine Learning in Medical Research
Machine learning has shown great potential in revolutionizing medical research, and the future looks promising. Here are some details about the future of machine learning in medical research:
- Advancements in Natural Language Processing: Natural language processing (NLP) is an area of machine learning that enables computers to analyze, understand, and generate human language. With advancements in NLP, it will become easier to extract meaningful insights from large amounts of unstructured data, such as medical journals, clinical notes, and patient feedback.
- Development of New Algorithms: Machine learning algorithms are constantly evolving, and new algorithms are being developed that can handle more complex tasks. For example, deep learning algorithms are being developed that can analyze medical images with greater accuracy than traditional machine learning algorithms.
- Integration with Other Technologies: Machine learning is being integrated with other technologies, such as robotics and virtual reality, to create new medical applications. For example, machine learning algorithms can be used to analyze the movement patterns of patients with neurological disorders, and this information can be used to develop more effective rehabilitation programs.
- Ethical Considerations: As machine learning becomes more prevalent in medical research, it is essential to consider the ethical implications of its use. For example, there are concerns about data privacy, bias in algorithms, and the use of machine learning to make life-and-death decisions. It is essential for researchers to address these concerns and develop ethical guidelines for the use of machine learning in medical research.
Overall, the future of machine learning in medical research looks promising, with advancements in natural language processing, the development of new algorithms, integration with other technologies, and ethical considerations all playing a role in shaping its development.
In conclusion, machine learning is transforming the landscape of medical research and has the potential to improve patient outcomes significantly. Despite the challenges and limitations, the future of machine learning in medical research looks promising with advancements in natural language processing, algorithm development, and integration with other technologies.
For researchers looking to harness the power of machine learning, California Academics offers medical research paper writing services that can assist with preparing research proposals, conducting literature reviews, and writing research papers. By leveraging the expertise of professional writers, researchers can save time and resources and ensure their findings are presented in a clear and concise manner.